目視チェックが大変な画像をPythonを利用して、同一画像や類似画像を検索する方法のご紹介です。
画像類似度の抽出方法がわかれば、似たような画像をグループとしてまとめる方法も可能です。参考に階層指定クラスタリングのPythonコード紹介と活用例の記事もございますので、御覧くださいませ。
Phashを用いた画像類似度判定方法を解説
ライブラリのインストール
import imagehash
from PIL import Image
ライブラリのインストールがまだの方は下記コマンドでインストール
pip install imagehash
pip install pillow
pipでのインストールでができない方はPythonでpip installが使えない時のプロキシとSSL無視をご覧くださいませ。
phashで画像の特徴を抽出する
ここでは2つの画像(つけ麺、つけ麺スープのみ)を用いて解説致します。
image_file_1
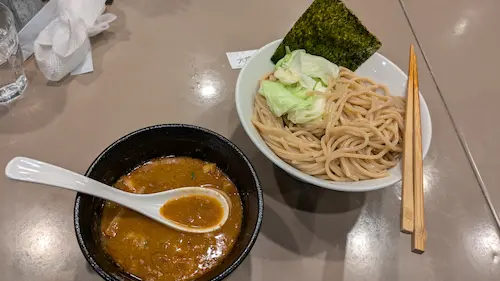
image_file_2
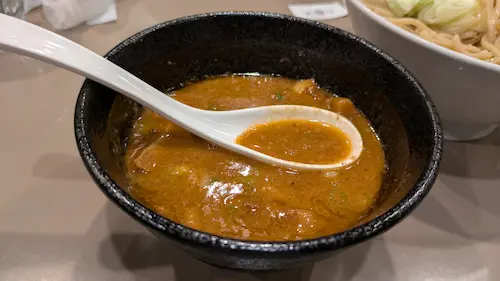
image_file_1 = r"C:\Users\abi00\OneDrive\デスクトップ\gazou\tukemen1.jpg"
phash_1=imagehash.phash(Image.open(image_file_1))
phash_1
変数phash_1に画像の特徴が格納されます。
#結果
array([[ True, False, True, True, True, True, False, False],
[ True, True, False, False, False, False, False, True],
[ True, True, True, False, True, True, False, False],
[False, True, True, False, False, True, False, False],
[False, False, True, True, True, False, False, False],
[ True, True, False, False, False, True, True, False],
[ True, False, False, False, False, True, True, True],
[ True, False, True, True, True, False, True, False]])
画像類似度判定:同一画像検知は引き算結果が0のものを抽出する
phashで抽出した値は引き算することで類似度を出すことができます。同じ画像で引き算すると値は0になります。同一画像を見つけたい場合の目安になります。この手法は精度が高いものですが、同じ画像を元に片方の画像のみに機械的に文字を入れて比較しても0と出るケースがあります。100%の精度ではないので一部運用でカバーする必要があります。
image_file_1 = r"C:\Users\abi00\OneDrive\デスクトップ\gazou\tukemen1.jpg"
image_file_2 = r"C:\Users\abi00\OneDrive\デスクトップ\gazou\tukemen1.jpg"
phash_1=imagehash.phash(Image.open(image_file_1))
phash_2=imagehash.phash(Image.open(image_file_2))
phash_1-phash_2
#結果
#0
画像類似度判定:類似度でどのくらい違う画像かを算出する
次は違う画像ファイル(つけ麺、つけ麵スープのみ)で引き算してみると差異が30になり、違う画像とはっきり認識されています。0から距離が離れるほど画像は似ていないことになります。
image_file_1 = r"C:\Users\abi00\OneDrive\デスクトップ\gazou\tukemen1.jpg"
image_file_2 = r"C:\Users\abi00\OneDrive\デスクトップ\gazou\tukemen2.jpg
phash_1=imagehash.phash(Image.open(image_file_1))
phash_2=imagehash.phash(Image.open(image_file_2))
phash_1-phash_2
#結果
#30
似た手法でaverage_hashとdhashがある
hashには様々な種類があり、Pythonではimagehash.average_hashやimagehash.dhashを簡単に利用できます。実際の画像を用いて比較して、どの手法が適しているかを試すことをおすすめいたします。コード例は下記です。
【average_hashの例】
image_file_1 = r"C:\Users\abi00\OneDrive\デスクトップ\gazou\tukemen1.jpg"
image_file_2 = r"C:\Users\abi00\OneDrive\デスクトップ\gazou\tukemen2.jpg"
phash_1=imagehash.average_hash(Image.open(image_file_1))
phash_2=imagehash.average_hash(Image.open(image_file_2))
phash_1-phash_2
#結果
#33
【dhashの例】
image_file_1 = r"C:\Users\abi00\OneDrive\デスクトップ\gazou\tukemen1.jpg"
image_file_2 = r"C:\Users\abi00\OneDrive\デスクトップ\gazou\tukemen2.jpg"
phash_1=imagehash.dhash(Image.open(image_file_1))
phash_2=imagehash.dhash(Image.open(image_file_2))
phash_1-phash_2
#結果
#33
phashと比較すると30から33に類似度が変わりました。
Akaze特徴量を用いた画像類似度判定方法を解説
ライブラリのインストール
Phashのライブラリに加えて下記を呼び出します。
import numpy as np
import cv2 as cv
import gc
OpenCvに読み込むために画像形式を変換する
画像系ライブラリのOpenCvで画像ファイルを認識するために形式を変換します。
#画像形式変換(pilowsからopencv)
def change_pilow_cv2(image_fille):
image_2 = np.array(image_fille,dtype=np.uint8)
if image_2.ndim == 2:#モノクロ画像
pass
elif image_2.shape[2] ==3:#カラー
image_2 = cv.cvtColor(image_2,cv.COLOR_RGB2BGR)
elif image_2.shape[2] ==4:#透過
image_2 = cv.cvtColor(image_2,cv.COLOR_RGBA2BRRA)
return image_2
Akaze特徴量を抽出する関数
#akaze特徴を抽出
def akaze_(image_file):
image_1 = change_pilow_cv2(image_file)
#akazeロジック
akaze = cv.AKAZE_create()
kp,des = akaze.detectAndCompute(image_1,None)
del image_1
del akaze
del kp
gc.collect()
return des
上記のimage_1画像ファイルを取り込み実施してみます。下記のように特徴量が抽出されます。
akaze_(Image.open(image_file_1))
#結果
array([[ 1, 2, 14, ..., 253, 255, 55],
[ 37, 230, 77, ..., 127, 179, 32],
[101, 237, 205, ..., 62, 34, 1],
...,
[ 97, 189, 76, ..., 80, 255, 39],
[ 65, 249, 13, ..., 3, 176, 53],
[ 1, 134, 255, ..., 113, 255, 63]], shape=(9292, 61), dtype=uint8)
Akaze特徴量で抽出した値を比較して類似度を出す関数
Akazeモデルで抽出した2つの画像特徴を比較して類似度を出す関数です。
#距離を比較
def comparison(akaze_1,akaze_2):
bf = cv.BFMatcher(cv.NORM_HAMMING,crossCheck=True)
maches = bf.match(akaze_1,akaze_2)
distan = [m.distance for m in maches]
ret = sum(distan) / len(distan)
return ret
同じ画像ファイルであれば距離は0。
a=akaze_(Image.open(image_file_1))
b=akaze_(Image.open(image_file_1))
comparison(a,b)
#結果
#0
異なる画像をインプットすると類似度が数字で出ます。phashと同じく0から遠いほど類似度が離れています。
a=akaze_(Image.open(image_file_1))
b=akaze_(Image.open(image_file_2))
comparison(a,b)
#結果
#88.24245196706313
画像類似度を判定するアンサンブルモデル
今回はhashとakazeをメインに画像特徴量を抽出した類似度判定をご紹介しました。これらのモデルを組み合わせたアンサンブルモデルを構築し、更に精度を上げる方法もあります。1つのモデルで実現したいことが叶わない時に複数モデルを組み合わせて数学計算してあげると精度UPが実現できます。